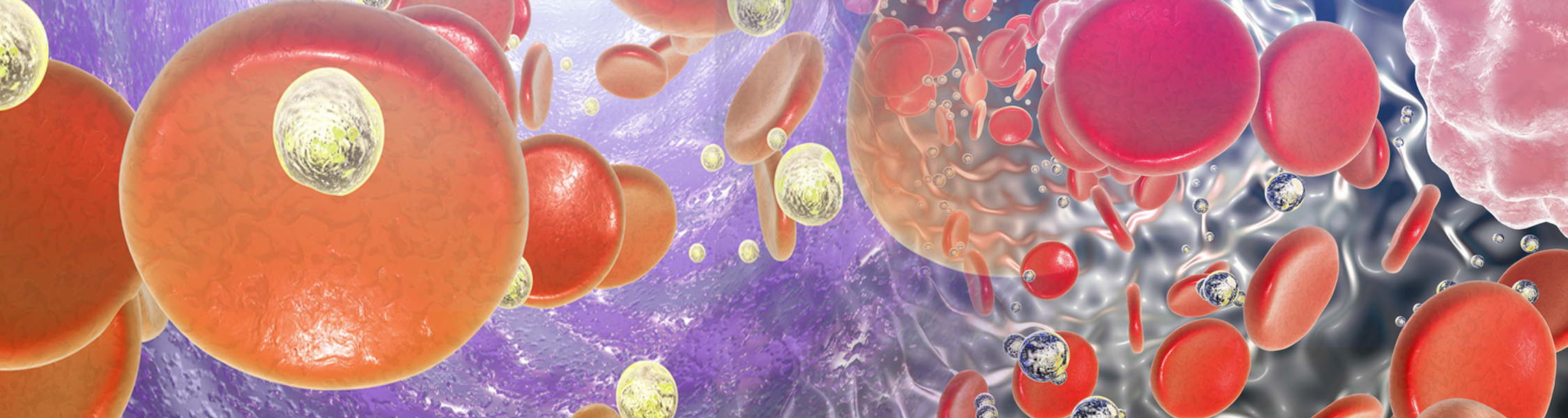
Rahim Zahedi1*, Iman Malek2, Reza Eskandarpanah2, Amir Mohammad Mirzaei3, Omid Noudeh Farahani4 and Nima Rezaei2
Received: August 10,2022 Published: August 24,2022
Corresponding author: Rahim Zahedi, Department of Renewable Energy and Environmental Engineering, University of Tehran, Tehran, Iran
DOI: 10.32474/JBRS.2022.02.000127
The present paper offers a method in sizing a combined battery and PV system connected to the power grid with the aim of providing load during peak consumption. PV and battery systems, which are used today in many residential, official and commercial sectors around the world, are often connected to the national power grid and PV and battery systems are considered as backup. The most important function of such a system, apart from supplying electricity during a power outage, is to minimize the cost of electricity consumption by reducing power consumption during peak grid loads. Initially, the load required by the home sector was simulated using Homer software. Based on the peak load time announced by the National Electricity Grid of Iran, the amount of load consumed during the peak time is obtained and the maximum amount of electricity consumed during the peak in different months is equal to the capacity of the PV system and battery. Since the electricity supplied by this system is the most expensive type of electricity consumed, the use of PV system and battery is justified.
Keywords: Sizing; Homer software; Renewable energy; PV battery system
In today’s world, with the expansion of technology and industrial tools, as well as the need to supply energy needed by various industries, the use of new and renewable energy has become inevitable. Among these, solar energy has a special place, and the use of this energy is increasing rapidly [1]. Meanwhile, the use of solar energy due to uncertainties in power generation (lack of uniform solar radiation and climatic and regional conditions, exact mismatch between production time and electricity consumption, etc.) has less reliability than energy supply from fuel Fossil fuels, which means the existence of batteries and electrical energy storage devices makes it necessary [2].On the other hand, the high initial purchase and installation cost of batteries and photovoltaic panels requires us to evaluate and carefully examine the required power and “sizing” the batteries and photovoltaic panels to provide the required energy at the lowest possible cost [3]. Many research groups have carried out PV and battery sizing optimization [4]. Currently, often constant electricity tariff or average load & PV data of the chosen sites are used as input data. Many of this research do not consider the interaction between variable electricity prices and the optimal battery and PV sizes.
Von Appen et al. [5] introduced an approach based on a mixed integer linear program. They use yearly load energy consumption from all analyzed households, which leads to a more general optimization for all households. However, the electricity consumption profile varies for different houses. The optimized results may be hard to be met by individual customer requirement. Recently, David Parra et al. [6] present a method to obtain the optimum community energy storage systems for end user applications. The same problem as before, they used constant electricity price and pre-defined battery charge and discharge operation. In this article, after examining the various methods of sizing photovoltaic panels and batteries, a method for sizing batteries and photovoltaic panels is proposed, which is obtained by using the amount of electricity consumed by a residential house during the peak load of the national electricity grid. In this method, the desired residential, commercial, office or industrial sector is connected to the national electricity grid (Figure 1) and the solar system serves as a backup system to generate power at peak grid load times. This minimizes the cost of electricity consumed by the unit.
Figure 1: Residential section connected to the national grid and equipped with batteries and photovoltaic panels.
Sizing methods are divided into six general categories. Rule of thumb, numerical, analytical, computer software, artificial intelligence, and hybrid methods [7]. These methods evolve over time and more accurately calculate the amount of power a consumer needs. The thumb method is the oldest sizing method and as its name suggests, it gives approximately the desired size [8]. The advantage of this method is its high speed and simple calculation, and its disadvantages are the significant error percentage in some cases, low reliability, and the need for more initial investment. In this method, sizing of photovoltaic panels is obtained by multiplying a safety factor Sf by the amount of power that provides the load. This coefficient is usually chosen based on the designer’s experience [9].
EL is the amount of energy consumed in the desired section, is the efficiency of the photovoltaic panel used, is the efficiency of the inverter and is the amount of solar radiation time. The equation provides the power required for the PV panel. In the numerical method, which is more advanced and accurate than the thumb method, the size of the panel and the battery are calculated by iterative solution (repeating new data at each stage until an acceptable answer is obtained) [10]. By calculating the technical parameters (such as the percentage of inability to supply load, duration of non-supply of load, etc.) and considering the minimum and maximum size for PV and batteries of this method, it gives the first and lowest acceptable power for PV and batteries [11]. In the analytical method, all battery sizes from the lowest to the highest value are tested for the desired panel size. If the required power is not provided, the size of the photovoltaic panel will be one step larger and all possible batteries will be selected again, and this cycle will continue until we reach the first appropriate answer [12].
In the analytical method, a graph of decision variables (here panel and battery size and technical parameters) is drawn according to each other and for all values, and the best panel and battery size is selected from these diagrams [13]. In this method, unlike numerical methods, panel and battery size is obtained at the same time and the minimum size is selected to meet the needs of the consumer. Nowadays, panel and battery sizing using computer software is the most used among different sizing methods and the reason for this is the high accuracy and convenience of these methods [14]. The most famous computer software that has significant performance in the field of sizing is HOMER software, which was designed and made available to the public by the US National Renewable Energy Laboratory. In this software, by entering home load data and types of photovoltaic panel sizes and available batteries, as well as connecting or not connecting of desired section to the national grid, the software, in order of priority, provides the best combination and size of systems as directory for the user.
Artificial intelligence methods used in sizing are divided into 4 general groups (Figure 2). In all these methods, the initial population is generated and then, using the evaluations performed, the values of the target functions are improved to approach the desired response. In this paper, contrary to the methods mentioned that only the electrical load consumed by the unit was calculated and the appropriate capacity to supply the load was determined, the load profile of the national power grid is also considered. First, the peak load time of the power grid is determined and then the electrical load consumed by the unit at the peak grid consumption is calculated to explain the capacity of the system in such a way that it responds to the desired house during the peak load time.
In this paper, the electrical load of a residential house is measured in one month and then simulated using HOMER software for the whole year. Also, the average consumption of days of a month has been selected as daily consumption in that month and changes in consumption between days of a month have been omitted. The average daily consumption of the house is approximately 10 kWh, and the maximum is up to 15 kWh. How to consume electricity at different times of the day is shown in Figure 3. Electricity consumption tariff in Iran is different at different times of the day [15]. The start and end time of the peak load of the grid according to the announcement of the Ministry of Energy in the months of July of 2020 to March of 2021 is as shown in Figure 4. This table is obtained from the information of Iran Energy Exchange. Complete information on peak load, medium load and low load is also provided. The method proposed in this paper is to use PV system and battery as backup system to supply power consumption during peak grid load to minimize power costs. Sizing such a system requires knowing the load consumption of the house during the peak load of the global power grid.
By simulating the consumption load of the desired house in 12 months of the year according to the previous part, the consumption load of the house can be obtained. The house in question is located in Tehran with a longitude of 35.68 and a latitude of 51.38. Figure 5 shows the grid load times for different months of the year in red lines. Also, for the first three months of the year, which is not in the figure and table, the load is considered according to the month of March. By calculating the level below the chart, the amount of electricity consumption of the house from the beginning to the end of the peak grid load in different months has been calculated. It should be noted that the peak load of the house does not necessarily correspond to the peak load of the electricity grid, and the information extracted from the peak hours of the national electricity grid is used only in the pricing of electricity consumption of the house. Home consumption culture can be matched to the average consumption of the community (peak consumption at peak grid consumption) or considered different. The house in question has operated according to the grid in some months and differently in some months.
Different scenarios can be defined for sizing PV systems and batteries with this method. By calculating the cost for different capacities of PV system and battery, it is possible to choose the capacity that provides the most benefit to the consumer in proportion to the cost by providing a part of consumption, but in this article, full supply of electricity load during peak consumption is considered [16]. In order to fully supply the electricity consumption of the house during the peak load, it is necessary to select the maximum consumption during the peak load in different months. As shown in Figure 5, the maximum area obtained is 4.12 kWh, which is selected as the desired size to fully supply the household load at peak times. Considering the average of 5 hours of sunshine per day, the capacity for PV is 800 KW and for the battery it is exactly equal to the maximum consumption of about 4.12 KWh.
In this article, after introducing sizing as the first and basic step of installing and equipping PV systems and batteries, its various methods were introduced and the uses of each in different parts of the world were examined. In the next step, the time of arrival of the national grid to the peak load in different months of the year was determined. Then, the electrical load of a building was simulated using HOMER software in the twelve months of the year and the consumption was determined at different hours of the day. In order to minimize the cost of electricity consumption for the desired house, it was proposed that the PV system and the battery supply the electricity of the desired house only at peak times when the price of electricity consumption is at its most expensive, because the complete power supply of the desired unit from the PV system and the battery requires a lot of initial cost. The proposed method for sizing without the need for high investment costs. In the most efficient case, all the electrical needs of the house in question during the peak load of the grid from the PV system and battery supply and electricity costs are greatly reduced.
a) Proposing a method for sizing batteries and photovoltaic panels, which is obtained by using the amount of electricity consumed by a residential house during the peak load of the national electricity grid.
b) Minimizing the cost of electricity consumed by the unit.
c) Simulating the load required by the home sector using Homer software.
Bio chemistry
University of Texas Medical Branch, USADepartment of Criminal Justice
Liberty University, USADepartment of Psychiatry
University of Kentucky, USADepartment of Medicine
Gally International Biomedical Research & Consulting LLC, USADepartment of Urbanisation and Agricultural
Montreal university, USAOral & Maxillofacial Pathology
New York University, USAGastroenterology and Hepatology
University of Alabama, UKDepartment of Medicine
Universities of Bradford, UKOncology
Circulogene Theranostics, EnglandRadiation Chemistry
National University of Mexico, USAAnalytical Chemistry
Wentworth Institute of Technology, USAMinimally Invasive Surgery
Mercer University school of Medicine, USAPediatric Dentistry
University of Athens , GreeceThe annual scholar awards from Lupine Publishers honor a selected number Read More...